How do we act on Educational Data?
Overview
This chapter covers the unique affordances that educational data and learning analytics can give us in our efforts to actually make data-informed decisions and act upon this data to improve teaching and learning.
Why is this important?
It’s all well and good to engage in work that gives us information about our learners, such as their assessment scores, evaluations of learning tasks, classes etc., as well as other educational data, but how do we interpret and act upon this information? With a key phrase tied to LA – that it should provide us with ‘actionable insights’ – its important to look different ways to think about these actions and what they c
Learning Objectives
- Understand the challenges in the interpretation of data
- Understand how data may be acted upon
- Consider how to ensure these actions are viable
Guiding Questions
As you’re reading through these materials, please consider the following questions, and take notes to ensure you understand their answers as you go.
- What is the most effective way of presenting lots of information visually? How do you know how to read it? Did you need help learning this skill?
- When you see information related to our work or study, do you innately know how to act upon to improve?
Key Readings
Corrin, L.(2021). Mind the Pedagogical ‘Gap’ when preparing staff to use learning analytics. NEXUS. Retrieved from https://www.solaresearch.org/2021/06/mind-the-pedagogical-gap-when-preparing-staff-to-use-learning-analytics/ on August 10, 2021.
Corrin, L., & De Barba, P. (2014). Exploring students’ interpretation of feedback delivered through learning analytics dashboards. In 31st Annual Conference of the Australian Society for Computers in Tertiary Education (ASCILITE 2014),’Rhetoric and Reality: Critical perspectives on educational technology’, Dunedin, New Zealand, 23-26 November 2014/B. Hegarty, J. McDonald and SK Loke (eds.) (pp. 629-633).
Lockyer, L., Heathcote, E., & Dawson, S. (2013). Informing Pedagogical Action. American Behavioral Scientist, 57(10), 1439–1459. https://doi.org/10.1177/0002764213479367
Wise, A. F., & Vytasek, J. (2017). Learning analytics implementation design. Handbook of learning analytics, 1, 151-160.
Back to our question…
Given that any solid inquiry starts with a question or questions, we should really think about the purpose of what we’re doing. If we are evaluating something small, like the use of a youtube video for learning, or something large like how to increase engagement in an AR experience across an entire school, the questions we ask should be specific to that context and the need to find an answer.
Validity and Reliability
When it comes to asking questions about assessment or evaluation strategies, we need to look at their validity and reliability. Both of these ways to think about assessment (and evaluation for that matter) take a critical stance towards the design of these practices, by asking questions about the purpose and delivery of different tasks we ask learners to engage with.
Depending on the subject area being taught and assessed, validity and reliability may not be something we need to dig too deeply in, but for others that use exams and other forms of knowledge testing (such as health sciences and medicine), it’s quite common. Practices around validity and reliability often overlap with those of data science and learning analytics because they do involve educational data and the analysis of this data. Downing (2003) presents an in-depth discussion of this for medical education given that most assessments in this space require evidence of validity.
Reliability
This refers to the extent to which something consistently and accurately measures learning
Reliability is usually examined from the viewpoint of providing consistent deployment strategies, such as all students having the same timeframe to complete assessments as well as the same instructions, supports and marking criteria. Ideally all students should come to an assessment with a similar basis of prior knowledge, and the assessment. With these and other factors in place, we can assume that the outputs we get from learners will be consistent as well, meaning the range of successful completion measures (marks) of these tasks will be consistent across time.
For evaluation projects, we can also look to ensure consistency through how evaluation methods are deployed, what is involved in completing any surveys or interviews, and the data we ultimately get from the evaluation.
Validity
This refers to the extent to which it measures what it intends to measure, without ‘contamination’ from other factors.
For example, if a student is to be assessed on an analysis of literature through a video they produce, and they don’t have that much experience in video production, their work on the analysis may be confounded by putting lots of effort into learning about making videos something that doesn’t have anything to literature.
There are many types of validity, with different researchers describing how each can be applied.
- Content validity – Does the assessment content cover what you want to assess (e.g., knowledge, skills, abilities and attitudes)?
- Consequential validity – Does the assessment achieve the purposes for which it was created? In other words, if most assessments are designed to ensure that students can demonstrate evidence that they’ve learned X, Y or Z, what is it about the task that may result in not everyone being successful?
- Construct validity – Does the assessment design actually measuring what it’s supposed to measure?
Other types of validity to think about are as follows:
- Face validity – Does the assessment and what’s included in it appear to be appropriate for target learners?
- Criterion-related validity – How well does the assessment measure what you want it to? Think about this as Construct validity, but and extended and deeper look at what an assessment is measuring.
For more on validity, check out Sobart’s paper (2009).
Interpreting Data
If you’ve ever explored sample educational data before, it is sometimes a challenge to wade through. Understanding what each column actually means based on some abstract naming conventions generated by a system or a researcher, it can take time and energy to scour through and actually make meaning of it all.
The interpretation of data is thus a really important factor to consider when engaging with Learning Analytics, because the data and the presentation of data can vary wildly with some bad examples being referred to as a #chartcrime on professional networking sites.
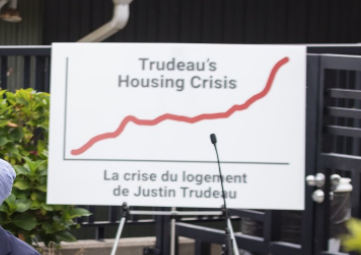
As Corrin (2021) discusses, the use of visualisations for Learning Analytics may excite many educators due to their potentially beautiful designs and use of colour, however the meaning that they convey may not be entirely clear to those who are the intended audience. This means that the type of visualisation or chart chosen should convey the information in a way that makes it very clear what the chart is communicating in the most objective way possible. Have a look at the example visualisations from the previous chapter again – is it clear what they mean? Could they be presented differently or with more information added to make them clearer? As LA and LA visualisations become more commonplace, with some institutions starting to adopt LA dashboards for students, this is ain important issue to consider.
Given that many of those viewing these visualisations may have played no role in collecting the data or making decisions around visualisations, they simply don’t have the contextual knowledge to make meaning of what hey are seeing, and if they misinterpret it, this could be damaging to teaching and learning.
Is what we’re seeing valid and reliable?
One question we need to ask ourselves is about the nature of the data – is it valid and is it reliable? In one such example, a common data point collected for educational data is a student’s IP Address – the unique identifier given to their computer by their Internet Service Provider (ISP). Using this data it’s possible to estimate (which pretty decent accuracy) where a computer is located in the world. Feel free to try it yourself using WhatismyIPAddress. The challenge here is that many people use a service called a VPN or Virtual Private Network, that allowers internet users to mask their true location in order to protect their privacy, so if I want to determine where my students are geographically located, I honestly don’t know who is using a VPN and who isn’t, so it would be more reliable to simply ask.
Another common example that comes up in learning analytics research is the concept of ‘time on task’, which describes how long a student looks at learning materials or works on an assignment or activity. Given that most educational systems only give us the exact time when a resource was accessed, the time that students spend engaging with something is not reliable, as they may open a web page, go and watch a movie on the couch for 3 hours then come back and finish reading the page.
The Self-fulfilling Prophecy for Teachers and Students
Archer and Prinsloo (2020) and Dietz-Uhler and Hurn (2013) speak about a common concern in the application, interpretation and use of learning analytics to drive action. One has to do with teacher bias, in that if a teacher sees educational data from individual students, this may drive to initiate or reinforce bias towards that individual student, causing knock-on effects that may influence grading / marking and how the student in perceived. Additionally, when students engage with their own learning analytics, they may come to take different approaches. For example, if a student is shown that their mark is below average or that they engage with the class less than the average student in their class, they can either use this to motivate themselves to improve and engage more, or if they already have a deficit mindset towards themselves, this data will reinforce this self-bias and their success in the class may be reduced further.
Its important then to be mindful of both the data we collect, why we’re collecting it, how we display it, who we display it to and for what purpose.
Self Regulation
An incredibly productive area of educational research for the last 30 years has been on Self-Regulation or Self-Regulated Learning (SRL). This area of research explores how learners monitor and act upon their own learning processes, including metacognitive activities like reflecting on their own abilities and adjusting their strategies based on their own self-understanding. Many studies in this area are now leveraging technologies to explore study strategies in students, and even learning analytics.
If you’re interested in this aspect of learning, a study by Pardo, Han and Ellis (2016) outline how the researchers measured self-regulation and different instructional interventions and prompts through learning analytics data and what this could mean for teachers and for learners. Given that learning is a much more nuanced process than reading and taking a test or writing an essay, how students navigate their own strengths and limitations as come to self-understandings about their learning, this is a very exciting way to think about learning analytics.
Acting upon Data
So the question becomes, how can we reliably and responsibly act upon data to support our pedagogical or evaluation aims? Lockyer, Heathcote and Dawson (2013) outline different types of analytics that are intended to inform pedagogical action, namely:
- Checkpoint Analytics: Analytics that are essentially a status update on the learner, including behavioural information related to their engagement with a learning platform. This can then lead to automated or instructor generated reminders and other encouragement techniques.
- Process Analytics: This provides instructors with insights into the process by which students go about completing a task. If they’re working together online, then perhaps data related to their engagement would work, or if they’re working on a large project, it’s the time they spend reading and researching combined with their submissions.
By thinking about who has access to different analytics and their visualisations, then thinking about what we can do with this data to further support learning is a really important step in designing a learning analytics approach. This is why much of the built in learning analytics that we see in an LMS / VLE these days is usually instructor-facing and tied to Checkpoint Analytics. These are quite easy to interpret and are pretty easy to deduce action from – a student hasn’t logged in to an online class for over a week? Maybe send them an email. A student hasn’t looked at key readings that are essential for completing an assignment? Let them know.
One consideration is that if we’re wishing to evaluate the effectiveness of a piece of learning content or how easily students understood the instructions of an assignment, it’s probably better to aggregate data and move away from individual data points so that individuals then become anonymous and all we can see are the trends and patterns related to our original questions.
As process analytics tell us more about the how of student learning, another difference between these types of analytics are when we’re able to act upon them. For checkpoint analytics we may be able to act on them immediately, whereas for process analytics, we may need to wait until the process is complete (e.g., the design of a group project) before we can act to make changes on it as making changes ‘mid-stream’ may disrupt the student experience.
When it comes to students viewing their own Learning Analytics, this is where this will be up to the student to act, however they should probably be given some sort of guidance on how to interpret their own data, what it means and appropriate ways to act based on what they see. Students are no different than educators in this regard – everyone will most likely need some training on how to act upon the data they have access to. Again, to avoid self-fulling prophecies, aggregate data may also be used for student-facing learning analytics and dashboards so that whole-group data can serve as prompt for students to self-reflect on what they’ve been doing and how they’ve been doing it, as opposed to a mechanism for self-judgement.
So Which Action is the right Action?
If the questions related to our implementation and use of learning analytics are clearly articulated and help us to answer these questions, then derived actions should be clear and we can be confident in our actions, but if our questions are vague then our actions will be harder to interpret.
Ultimately here is no universal ‘correct’ way to interpret and act upon educational data, so the clarity around our questions and our intentions is what matters. Regardless, as long as we are considering the possible effects of our actions on our students, as well as on ourselves, we’ll then be in a better position to try interventions that will meaningfully improve learning.
Below are a few examples:
- If we are evaluating the value of a youtube video we put in our class and find that no one has viewed it, or they only watch the first few seconds then leave it, we can assume that it may not have been engaging enough to continue watching, so we should pick another video.
- If we find that students aren’t very successful on an assignment as evidenced through their marks / grades, but we also see that the assignment instructions are buried under 5 clicks of navigation in the LMS / VLE, then we can move them to make them easier to access.
- If students aren’t interacting that much in a discussion forum, and we revisit the question and it says “Who discovered australia? Discuss”, maybe this isn’t the best prompt for encouraging a conversation.
Key Take-Aways
- The Interpretation of data and data visualisations is very subjective, so its design and how educators are trained on what it means is really important
- We also need to make sure that the data we have is actually measuring what we think it does, and is thus viable and reliable.
- As with research, we also have to understand that when our students and other teachers see data, this can influence their behaviour in a way that may hinder our goal of improvement.
- When we want to act upon data based on our understanding of the data and the insights we’ve gained from it, we need to think about the effects of our actions and how they may influence our learners and even ourselves.
Revisit Guiding Questions
Think about what you’ve learned here. Have a look at the questions you’re asking and their specificity. If you’ve explored some data that may be related to these questions, does your data answer the questions clearly, and provide a pathway to action? If not, have a think about alternative questions you could ask that might yield clearer interventions.
Conclusion / Next Steps
In this chapter, we discussed different ways in which we can take educational data and visualisations and think of ways to act upon them. Though there is no magic formula for doing so, there are a few different ways to think about it. In the next chapter we’ll look at the ethical issues that may arise from Assessment, Evaluation and Learning Analytics and how we can manage or mitigate them.
References
Archer, E., & Prinsloo, P. (2020). Speaking the unspoken in learning analytics: troubling the defaults. Assessment & Evaluation in Higher Education, 45(6), 888-900.
Dietz-Uhler, B., & Hurn, J. E. (2013). Using learning analytics to predict (and improve) student success: A faculty perspective. Journal of interactive online learning, 12(1), 17-26.
Downing, S. M. (2003). Validity: on the meaningful interpretation of assessment data. Medical education, 37(9), 830-837.
Jarke, J., & Macgilchrist, F. (2021). Dashboard stories: How narratives told by predictive analytics reconfigure roles, risk and sociality in education. Big Data & Society, 8(1), 20539517211025561.
Pardo, A., Han, F., & Ellis, R. A. (2016, April). Exploring the relation between self-regulation, online activities, and academic performance: A case study. In Proceedings of the Sixth International Conference on Learning Analytics & Knowledge (pp. 422-429).
Stobart, G. (2009). Determining validity in national curriculum assessments. Educational Research, 51(2), 161-179.
Further Reading
Reliability and Validity, New Zealand Ministry of Education
A shared language of assessment Principles from Strengthening Student Assessment, QUT
Did this chapter help you learn?
100% of 2 voters found this helpful.
Provide Feedback on this Chapter